by Ruohan Tang
A theoretical frame for an AI content-based footage retrieval system
Automatic video editing is one of the emerging trends in the film industry and film production studies, aiming to provide a solution to simplify the post-production workflow and improve the overall efficiency of film production.
In the current documentary post-production workflow, editors are often submerged in a large amount of raw footage obtained from the filming site. Because of the unpredictability in documentary production, editors need to review every single shot at the pre-editing stage to build up a footage library for the rough-cut stage. To complete a storyline within a coherent spatial and temporal paradigm, the process of selecting material manually becomes an essential part of the post-production workflow. Documentary productions built over a long filming process make for an enormous workload. Besides that, because editors are not usually involved in the filming process, directors are required to engage fully in the post-production workflow to review, select and reconstruct all footage with editors from pre-editing to the final cut. Thus, for a documentary project with a limited production budget, this time-consuming process at the pre-editing stage will have a corresponding side effect not only on efficiency but also on cost.
Drawing on some existing studies on AI (Artificial Intelligence) engagement in the video indexing and query system, I propose a theoretical framework for an AI content-based footage retrieval system based on attribute capture, vector–matrix transformation, intelligent algorithmic database classification and database retrieval. In a further discussion, I will argue how such a system could simplify the current pre-editing workflow in the documentary industry.
Researchers have developed two directions for the AI editing system based on the interdisciplinary studies of graphical recognition and machine learning. Both research paths currently focus on highly structured amateur video editing. In other words, the current stage of AI editing research is based on short video projects with a small and coherent footage database, an explicit storyline, predetermined scripts and a detailed shot list. One of the representative programmes is the computational video editing system developed by Leake and her colleagues, which achieves the automatic editing of footage based on dialogue-driven scenes (Leake, Davis, Truong & Agrawala, 2017).
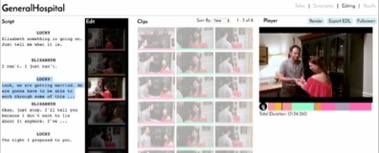
Figure 1. Computational video editing for dialogue-driven scenes developed by Leake et al.
Similarly, some researchers have been trying to develop a fully automated editing system utilising deep machine learning. They repeatedly train the machine to recognise the logic of film editing and montage and simulate the manual editing process based on pre-annotated data (Tsuchida, Fukayama & Goto, 2017; Taylor & Qureshi, 2016; Takemae, Otsuka & Yamato, 2005). This direction of research focuses on the segmentation and grouping of footages, as well as the recognition and arrangement of the corresponding clip elements under specific coding logic. Through such a process of machine learning, the AI editing system can edit the imported footages with constraints on the properties of the clip elements. For video projects with a relatively small database and a structural cinematic language, for example, music videos and YouTube videos, this type of research can improve the efficiency of their editing without human intervention; however, machine learning in acknowledging and arranging visual elements is still at the basic image-perception level. Thus, the final outputs of this fully automatic editing system are relatively monotonous and basic, and nowhere near the standard of an editor’s work.
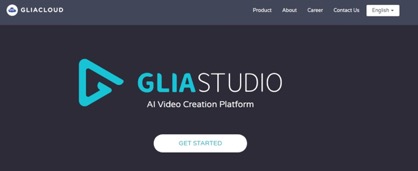
Figure 2. Gliacloud is an AI technology-based video editing software with editing packages to create videos with different versions for users to select from.
The second path is an editing system that combines AI support with human input (de Lima, Feijo, Furtado, Pozzer & Ciarlini, 2012; Wang, Mansfield, Hu & Collomosse, 2009). These AI editing developments are not designed to replace editors but rather assist them. By providing users with an interactive interface, this type of AI editing system works as an editing assistant to simplify the work of editors and preserve the space for human artistic creativity. First, the machine will, first, identify and segment video footage, and then process the raw material into specific effects according to the relevant instruction from users. For example, Adobe Premiere provides users with an AI plug-in to finish the colour-grading process of footage automatically. (Dove, 2019; Frazer, 2019)
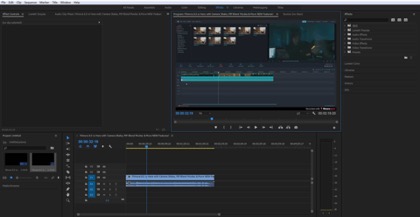
Figure 3. Premiere provides a Sensei AI platform to support users in matching the colour of footage automatically.
However, the exploration of AI engagement in documentary editing is still sporadic. The lack of research on automatic editing for documentary projects can be attributed to the following two reasons: the ‘firmness’ in the delineation of reality and unpredictability at the filming site. According to Bazin and Kracauer, who ‘saw the essence of cinema in the recording competence of film’ (Nebesio, 1999), the ‘filmness’ within the film medium is based on its mechanical reproduction of reality through photography. As the essentiality of perceptual reality, ‘spatial realism’ has been embedded into the depth of field shot and longshot (Bazin, 2014). However, the montage theory, which focuses on the event’s narrative, necessarily involves a great deal of space and time fragmentation, thus undermining perceptual reality (Bordwell, 1972). Unlike the montage theory, which focuses on the intervention of editing in constructing the single meaning from the individual frames to achieve an artistic creation, the realistic concept in filmmaking tries to represent the polytropic objective reality in frame with instantaneous and arbitrary nature (Kline, 2013). Thus, in practice, the documentary filmmakers who, following Bazin’s thinking on the representation of reality, try to emphasise the primitiveness inherent in the frame rather than the engagement of artificial techniques. Therefore, the editing process of a documentary normally deals with many realistic images with pluralistic characteristics. Although documentary directors specify the appropriate synopsis, storyline and treatment before filming, the footage they obtained at the shooting site is usually uncertain and spontaneous. In addition to this, the preference for long shots and innumerable footages further complications make machine learning in automatic documentary editing extremely difficult. Therefore, an editing system that combines AI support with human input seems like a feasible way to develop the AI engagement of editing in documentary projects.
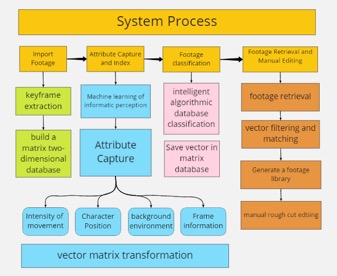
Figure 4. Theoretical framework of an AI content-based footage retrieval system process
Therefore, this study proposes a theoretical framework for organising a rough-cut footage of documentaries based on an AI content-based footage retrieval system, which could be regarded as a viable first step of the documentary’s automatic editing. In this system, sophisticated AI algorithms will be applied to capture attributes and index footages, thus facilitating post-production editing workflow. After importing the video data, a database of video information categories is created for an individual documentary project, and the machine will be trained with a specific identification model for informatic perception. A two-dimensional vector space is then created, and all the shots are placed as elements in a set. Meanwhile, keyframes of each footage are extracted and generated as a subset of the vector’s elements.
Then, based on AI neural networks, the system will achieve the attributes’ capture. The intensity of movement in the frame could be converted to a vector–matrix by calculating the distance of the spatial movement for the key points in two adjacent keyframes per unit of time. Such key points in the frame could be calculated and extracted by Lowe’s SIFT algorithm (Lowe, 2004). The subject’s position in the keyframe can be calculated through the motion tracking algorithm of the Kanade–Lucas–Tomasi feature tracker (Suhr, 2009) and further transferred into the vector–matrix. The attribute of the background environment in which the subject is filmed can be obtained by calculating the colour distribution of the colour histogram of a keyframe by the texture analysis algorithm the Gabor Filter (Young, Van Vliet & Van Ginkel, 2002). The frame’s general information can be annotated by encoding the frame with a difference–value Hash algorithm (Li, Deng & Xiao, 2011). This algorithm can be used to calculate the frame similarity in the subsequent retrieval process.
At the footage classification stage, the artificial neural system will initialise the training of interactive operations. With massive footage data, the artificial neural system gradually adapts to the specific image feature vector’s identification progression and further classifies and aggregates footages based on the attributes. After that, footages with similar feature vectors will be clustered into the defined area of two-dimensional space and saved as the matrix database for the user’s retrieval. When the user gives the command for retrieval for the specific content of the footages, the system will filter and match the vector–matrix that has been aggregated in the previous stage and generate the corresponding library for the documentary editor to efficiently complete the rough cut. This theoretical AI system could efficiently free directors and editors from the onerous process of reviewing and selecting the footage in the pre-editing stage of post-production. Such a method theoretically ensures that an extensive footage database can be pre-searched based on target information, which will save time on footage review in post-production and increase video editing efficiency while maintaining visual coherence. With help from this system, directors and editors can build storylines more quickly. Besides such an improvement of production efficiency, such a theoretical framework also provides an alternative way of developing AI editing systems based on in-depth learning on deconstructed symbolic cognition of camera language.
In the current editing process, editors interpret the meaning of each shot based on discrete symbols within the clips and construct the storyline with a highly structured left-to-right sequence. Thus, in developing cognitive science in the moving image perception, the researchers are trying to mimic this thinking process of editors in building up montage with symbolic structure in sequence. The machine has encountered a great challenge in recognising the symbolic representation without the structural database. Therefore, it has caused a suboptimal outcome in the development of automatic documentary editing. According to Deleuze’s viewpoint on cinematographic concepts, the cinematic language is not a universal language but ‘a composition of images and of signs, that is, a pre-verbal intelligible content (pure semiotics)’ (Deleuze, 1986). He also argued that the semiotics of Saussure’s linguistic tendencies in film study ‘abolishes the image and tends to dispense with the sign’ (Deleuze, 1986). Therefore, the theoretical frame I propose in this article has integrated Bordwell’s perspective on schematic cognition in film reading as the basis for machine learning on film cognition (Bordwell, 2012). This frame could also provide a deconstructed structural theoretical perspective on the cinematic language to develop automatic documentary editing.
Bibliography
Bazin, A. (2014). Bazin at work: major essays and reviews from the forties and fifties. Routledge.
Bordwell, D. (1972). The idea of montage in Soviet art and film. Cinema Journal, 11(2), pp.9-17.
Bordwell, D., & Carroll, N. (Eds.). (2012). Post-theory: Reconstructing film studies. University of Wisconsin Pres.
Deleuze, G. (1986). Cinema 1 The Movement- Image. trans,by. Habberjam, B. & Tomlinson, H., Minneapolis, MN: University of Minnesota.
de Lima, E. S., Feijo, B., Furtado, A. L., Pozzer, C., & Ciarlini, A. (2012). ‘Automatic Video Editing for Video-Based Interactive Storytelling’. 2012 IEEE International Conference on Multimedia and Expo, Multimedia and Expo (ICME), 2012 IEEE International Conference on, Multimedia and Expo, IEEE International Conference On (pp.806–811).
Dove, J. (2019). ‘Adobe Premiere Elements 2019: Movie Editor with an Ai Spin’. Macworld - Digital Edition, 36(1), pp.21–27.
Frazer, B. (2019). ‘Evolphin Adds Automatic Video Editing and Other AI-Driven Features to Zoom MAM’. StudioDaily. Retrieved July 17, 2019 from https://www.studiodaily.com/2019/07/evolphin-adds-automatic-video-editing-ai-driven-features-zoom-mam/
Jefferson Kline, T. (2013). ‘The Film Theories of Bazin and Epstein: Shadow Boxing in the Margins of the Real’. Paragraph, 36(1), pp.68-85.
Leake, M., Davis, A., Truong, A., & Agrawala, M. (2017). ‘Computational video editing for dialogue-driven scenes’. ACM Trans. Graph., 36(4), 130-1.
Li, Y., Deng, S., & Xiao, D. (2011). ‘A novel Hash algorithm construction based on chaotic neural network’. Neural Computing and Applications, 20(1), pp.133-141.
Lowe, D. G. (2004). ‘Distinctive image features from scale-invariant keypoints’. International journal of computer vision, 60(2), pp.91-110.
Nebesio, B.Y.(1999). ‘How to Account for Cinematic Experience?’, Film-Philosophy, 03(35).
Smoliar, S. W., & Zhang, H. (1994). ‘Content based video indexing and retrieval’. IEEE multimedia, 1(2), pp. 62-72.
Suhr, J. K. (2009). ‘Kanade-lucas-tomasi (klt) feature tracker’. Computer Vision (EEE6503), pp.9-18.
Takemae, Y., Otsuka, K., & Yamato, J. (2005). ‘Effects of Automatic Video Editing System Using Stereo-Based Head Tracking for Archiving Meetings’. 2005 IEEE International Conference on Multimedia and Expo, Multimedia and Expo, 2005. ICME 2005. IEEE International Conference on, Multimedia and Expo (pp.185–188).
Taylor, W., & Qureshi, F. Z. (2016). ‘Automatic video editing for sensor-rich videos’. 2016 IEEE Winter Conference on Applications of Computer Vision (WACV), Applications of Computer Vision (WACV), 2016 IEEE Winter Conference On (pp.1–9).
Tsuchida, S., Fukayama, S. & Goto, M. (2017).’Automatic system for editing dance videos recorded using multiple cameras’. in Cheok, A.D., Inami, M. & Romao, T.(ed).(2017). Advances in Computer Entertainment Technology. Heidelberg: Spriger (pp.671-688).
Wang, T., Mansfield, A., Hu, R. & Collomosse, J. P. (2009). ‘An Evolutionary Approach to Automatic Video Editing’. 2009 Conference for Visual Media Production, Visual Media Production, 2009. CVMP ’09. Conference For (pp.127–134).
Young, I. T., Van Vliet, L. J., & Van Ginkel, M. (2002). ‘Recursive gabor filtering’. IEEE Transactions on Signal Processi
Author Biography
Ruohan Tang, PhD Candidate at University of Southampton, Film Studies Department, within the China Scholarship Council (CSC) Arts Talent Program. His research focuses on space, memory and aesthetic value of Chinese Participatory Video. His research interests also include media technology, interactive visual media, immersive documentary and the technicalisation in the documentary industry. He is also working as the Training Officer in MeCCSA (The Media, Communication and Cultural Studies Association) PGN and has been listed into Future Talent Programme 2020-2021 in UK-China Film Collab as International Documentary Development Specialist.